1. INTRODUCTION
Copper is essential for oxygen reactions, metabolism and iron absorption in eukaryotes [1]. Tsvetkov et al. first identified a novel cell death mode that triggers cell death by directly binding to drive the accumulation of lipid-rich TCA cycle enzymes in mitochondria. The authors observed that increasing copper concentrations induced cell death, thus suggesting copper’s toxicity [2]. Under physiological conditions, mitochondria import copper through the carrier family protein SLC25A3 and store it in the matrix, where it is used for cytochrome C oxidase assembly; however, no known protein has been identified as a binder of copper [2, 3]. Copper has been found to promote proliferation, autophagy and lipolysis by binding non-catalytic sites of proteins [4]. In Wilson’s disease, ATP7B mutation results in excessive copper accumulation, formation of iron-sulfur clusters and decreased the activation of autophagy in hepatocytes [5]. Liver copper deficiency in patients with NAFLD increases steatosis severity, NASH development, and metabolic symptoms [6]. NAFLD forms spontaneously with lipid accumulation in rats deprived of dietary copper [7–9]. In addition, copper deficiency is closely associated with mitochondrial dysfunction and oxidative stress [10]. Decreased liver cellular copper content causes abnormal mitochondrial enlargement, thereby leading to mitochondrial dysfunction [11]. Copper deficiency in the body also leads to abnormal antioxidant function, thus resulting in an aberrant oxidative stress response. Interestingly, disrupted copper metabolism also induces abnormal iron metabolism in the liver and may potentially be associated with impaired functionality of iron transporters [12, 13]. Thus, cuproptosis has critical regulatory roles in numerous physiological and pathological processes.
HCC is among the top ten most common gross tumors and is the fourth leading cause of tumor-related death [14, 15]. Copper levels have been associated with liver cirrhosis and HCC; moreover, high copper content is associated with poor prognosis and elevated risk of HCC development. Infinite cell proliferation is a crucial hallmark of tumor cells [16–18]. In recent years, induction of programmed cell death in tumor cells has emerged as a potential therapeutic strategy for cancer treatment. The cuproptosis-related gene FDX1 enhances cuproptosis sensitivity by regulating protein lipoacylation [19]. Copper ions promote cell death through binding fatty acylated components and subsequently lead to abnormal accumulation of fatty acylated proteins, thereby highlighting the beneficial role of copper ions in metabolic diseases. However, the mechanism underlying hepatocyte cuproptosis remains inadequately investigated.
In our study, on the basis of transcriptome data and clinical features, we used an unsupervised clustering algorithm to identify the correlation between cuproptosis-related molecular subtypes and the characteristics of TME cell infiltration. Our findings highlight the major roles of cuproptosis-related molecular subtypes in TME regulation. Furthermore, we used a LASSO Cox regression model to identify genes associated with prognosis, and we constructed a prognostic model for HCC based on the cuproptosis-related risk score (CRRS). To quantify individual patterns of cuproptosis modification in patients with HCC, we developed a nomogram. Finally, we conducted gene cluster analysis and risk score evaluation to assess immune cell infiltration characteristics and immunotherapy sensitivity.
2. MATERIALS AND METHODS
2.1 Downloading and preprocessing of publicly available hepatocellular carcinoma datasets
Transcriptome expression data and survival information for patients with HCC were downloaded from The Cancer Genome Atlas (TCGA) and GEO (http://www.ncbi.nlm.nih.gov/geo/). Patients with HCC with incomplete clinical features were excluded. HCC data from the GEO database and TCGA–Hepatocellular Carcinoma data sets were collected for further analysis. For microarray data, we used the Affy and simplafty software packages to adjust background normalization and perform quantile normalization. HCC gene expression data sets (FPKM values) were downloaded from the Genomic Data Commons (https://portal.gdc.cancer.gov/), and the sva package was used to correct for bulk effects. Similarly, data on somatic mutations were obtained from the TCGA databases and analyzed in R (version 4.1) and the R Bioconductor packages.
2.2 Consensus clustering analysis of 19 cuproptosis-related gene regulators
Initially, 19 CRG regulators were obtained from the published literature and used to construct several CRG modification models. The 19 CRG regulators were NFE2L2, NLRP3, ATP7B, ATP1A, SLC31A1, FDX1, LIAS, LIPT1, LIPT2, DLD DLAT, PDHA1, PDHB, MTF1, GLS, CDKN2A, DBT, GCSH and DLST. Unsupervised clustering analysis was used to classify into two modification modes of CRGs, according to the optimal K value. Patient classification was performed with the ConsensClusterPlus R software package, and the stability of classification was ensured through 1000 cycle calculations. Overall survival (OS) among patients in different clusters was evaluated with the Kaplan-Meier method.
2.3 Identification of differentially expressed genes
We used the limma software package to compare the expression levels of 19 regulators of CRGs between patients with HCC and healthy individuals. The significance threshold for DEGs was set at |log FC|> 2, P < 0.05.
2.4 Construction of risk scores associated with cuproptosis
To further evaluate the potential prognostic value and molecular mechanisms of cuproptosis, we performed LASSO Cox regression to analyze the TCGA dataset by using the R glmnet package. The best prognostic biomarkers were screened from among the 19 CRG regulators. Subsequently, risk scores for OS and PFS were calculated separately by using the regression coefficients of the identified prognostic markers of CRGs. Patients were divided into low-risk and high-risk groups according to the median risk score, and their OS values was compared with the R ggsurvplot package in survival analysis. Additionally, we used the nearest-neighbor estimation method to estimate 1-, 3- and 5-year survival rates. The receiver operating characteristic curve was used to evaluate the prognostic value of the survival curve. To further validate the scientific validity of the CRG prognostic model, we used a training set comprising 259 and a test set comprising 258 HCC cases of 517 HCC cases from the TCGA and GSE76427 datasets. On the basis of the significant associations of CRGs with highly malignant and advanced HCC tumors, we constructed a nomogram through uniCox and multiCox analysis to independently analyze the prognostic reliability of the cuproptosis model in HCC and its relationships with clinical factors.
2.5 Comparison of TME cell infiltration
To comprehensively assess the extent of immune cell infiltration in HCC, we performed CIBERSORT analysis to estimate the compositions of immune cell types in HCC samples. We obtained gene sets representing 22 immune cell populations, including activated B cells, CD8 T cells, monocytes, dendritic cells resting T cells, macrophages and regulatory T cells. A single sample gene enrichment analysis was used to quantify the relative abundance of different immune cells in TME in each sample species and to assess associations with the CRGs.
2.6 Statistical analysis
T tests were used for the comparison of samples between groups, and one-way ANOVA was used to evaluate differences between three or more groups. Spearman’s correlation analysis was used to obtain the correlation coefficient between CRGs and TME immune infiltrating cells. The prognosis was analyzed through uniCox and multiCox regression analysis, and the survival curve was visualized with the Kaplan-Meier method. Chromosome CNV analysis of 19 CRGs was performed with the R RCircos package, and the mutation rates of the 19 CRGs were obtained with the R maftools package. P < 0.05 was considered significantly significant, and all data were analyzed in R software 4.1.
3. RESULTS
3.1 Genetic variation in cuproptosis-related genes in HCC
Recent studies have revealed 19 genes associated with CRGs: NFE2L2, NLRP3, ATP7B, ATP1A, SLC31A1, FDX1, LIAS, LIPT1, LIPT2, DLD DLAT, PDHA1, PDHB, MTF1, GLS, CDKN2A, DBT, GCSH and DLST. To investigate the genomic characterization of CRGs in patients with HCC, we evaluated somatic mutations and CNV in 371 HCC samples. The frequency of CRG mutations was approximately 10.24%, thereby indicating a relatively low mutation rate. Among the 19 CRGs, CDKN2A (3%) had the highest mutation frequency, and was followed by NFE2L2 (2%), NLRP3 (2%), ATP7A (1%), DLD (1%), MTF1 (1%) and DBT (1%), whereas the remaining CRGs did not exhibit any mutations ( Figure 1b ). In addition, the CNV analysis results indicated high deletion frequencies for MTF1, DBT, SLC31A1, PDHB, FDX1, DLST, DLAT, GCSH, ATP7B and CDKN2A ( Figure 1c ). Therefore, most CRGs tended to be co-deletions rather than co-amplifications with high CNV frequencies. The positions of CNV alterations on chromosomes are depicted in Figure 1d . Furthermore, we evaluated whether abnormal expression of CRGs might be associated with HCC malignancy by comparing mRNA expression levels in HCC and normal tissues, and identified 15 CRGs with significant differential expression patterns ( Figure 1a ). Moreover, Kaplan-Meier survival curves demonstrated a favorable prognosis associated with the 19 CRG regulators ( Figure S1 ).

(a) Differences in the expression of 19 CRGs between patients with HCC and healthy individuals. (b) Mutation frequencies of 19 CRGs in 371 HCC samples, detected with the R maftools package. (c) The CNV frequency of 19 CRGs in liver cancer. (d) Nineteen CRG expression sites on 24 chromosomes. Compared with normal tissues, *P < 0.05, **P < 0.01, ***P < 0.001.
3.2 Identification of cuproptosis subtypes in hepatocellular carcinoma
We investigated the clinical significance of CRGs in HCC through univariate Cox and correlation analysis. Nine CRGs (including LIPT1, DLAT and GLS) were significantly associated with negative prognosis in patients with HCC ( Figure 2a ). To further evaluate the role of CRGs, we used unsupervised clustering analysis to classify the expression levels of CRGs in the HCC cohort with complete survival information (TCGA and GSE76427). This analysis identified two distinct regulatory patterns, including 354 cases in CRG-associated cluster A and 163 cases in CRG-associated cluster B ( Figure 2b ). On the basis of the results of PCA, we divided 371 HCC samples into two distinct subtypes ( Figure 2c ). In addition, the survival rate of group A was significantly higher than that in group B (P < 0.05) ( Figure 2d ). We illustrated the relationship between clinical factors and gene expression of two different CRG patterns in heat maps ( Figure 2e ).

Construction of cuprotosis subtypes in HCC.
(a) A network of correlations including CRGs in TCGA cohort. (b) Consensus clustering matrix for k = 2. (c) Kaplan-Meier curves of OS for two cuprotosis subtypes constructed from 19 CRGs in patients with HCC. (d) Unsupervised clustering analysis of 19 CRGs in HCC.
3.3 Construction of cuproptosis phenotype-related differentially expressed gene clusters
To further investigate the potential regulatory role of CRG phenotypes, we obtained 4275 DEGs associated with CRGs with the R package Limma and divided them into three clusters according to unsupervised clustering analysis (gene clusters A–C) ( Figure 3a ), with 206 cases in gene cluster A, 256 cases in gene cluster B and 55 cases in gene cluster C ( Figure 3b ). Under this clustering algorithm, gene cluster B exhibited a favorable prognosis (P < 0.001) ( Figure 3d ). Interestingly, both gene clusters A and B belonged predominantly to cuproptosis cluster A, whereas patients with gene cluster C belonged to cuproptosis cluster B, which showed poor prognosis ( Figure 3c ). We observed significant differences in CRG expression among the three gene clusters, in agreement with the anticipated cuproptosis patterns ( Figure 3e ).

Prognosis and clinical characteristics of three cuproptosis gene clusters from patients with HCC.
(a) Venn diagram indicating genes shared by two cuproptosis subtypes. (b) Consensus matrix heat maps for three gene clusters, obtained with the R ConsensClusterPlus package. (c) Heat maps of clinical characteristics of three cuproptosis gene clusters. (d) Kaplan-Meier survival curve of the survival probabilities of patients with three gene clusters. (e) Expression of 19 CRGs in three gene clusters.
3.4 Prognostic value of cuproptosis-related signatures in HCC
To further investigate the prognostic value of cuproptosis, we developed a prognostic model based on data from 517 patients with HCC with complete survival information in the TCGA and GSE76427 datasets. CRGs with prognostic value were identified through LASSO Cox regression analysis. After establishing a LASSO Cox regression model with lambda minimization, we obtained seven CRGs with cuproptosis-related features and used them to construct a CRRS model ( Figure 4a, b ). The associations between the expression of seven CRGs and OS was illustrated with a forest plot. The expression levels of ARF4, HMGA1, PRKD1, TRIB3, ZIC2 and RPPH1 were significantly positively correlated with poor prognosis, whereas that of IL7R was significantly negatively correlated with good prognosis ( Figure 4c ). Differential expression levels of 19 CRGs were observed, whereas NLRP3, ATP7B, SLC31A1, DBT and GCSH did not show statistically significant differential expression. However, the remaining CRGs had significant effects on prognosis ( Figure 4d ). The correlations among cuproptosis-subtypes, gene clusters, CRRS and survival status were shown in a Sankey diagram. In the high-CRRS group, a greater proportion of patients experienced death than survived, whereas patients with low CRRS predominated in gene clusters B and C, and the proportion of gene clusters B and C was higher than that of gene cluster A in CRG cluster A ( Figure 4e ). In addition, the median score of CRG cluster B was significantly lower than that of CRG cluster A. Gene cluster C displayed a higher score than gene clusters A and B, and gene cluster B had the lowest median score ( Figure 4f ). The low-risk group and high-risk group were divided according to the median risk score. The low-risk group exhibited longer survival time than the high-risk group ( Figure 4g ), and the area under the receiver operating characteristic curve (AUC) values for 1-year, 3-year and 5-year OS were 0.731, 0.718 and 0.702, respectively ( Figure 4h ).
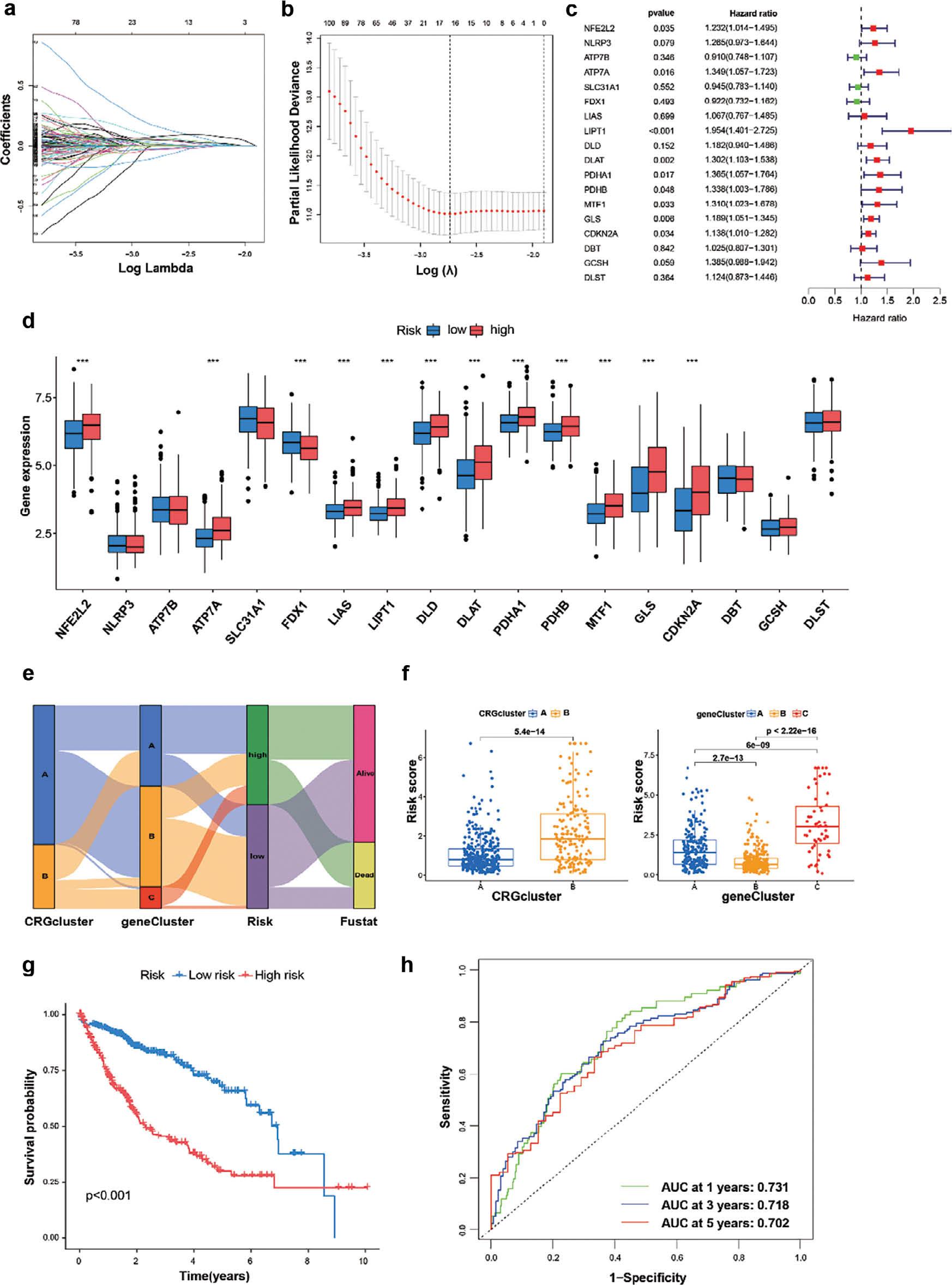
Determination of CRGs for prediction of HCC prognosis.
(a, b) LASSO regression analysis performed in the R glmnet package on 19 CRGs, showing the distribution of LASSO coefficients and partial likelihood deviance. (c) Forest plots of correlations between 19 CRGs and survival. (d) Expression of 19 CRGs in the high-risk and low-risk groups. (e) Sankey diagram showing the relationships among CRG clustering, gene clustering, risk coefficient and survival state. (f) Kruskal-Wallis test analysis of differences in risk scores between two CRG clusters and three gene clusters. (g) Kaplan-Meier curves showing survival in the high-risk and low-risk groups. (h) ROC analysis of CRGs and survival state.
3.5 CRGs are an independent prognostic indicator for patients with HCC
On the basis of the significant association of CRGs with highly malignant and advanced HCC tumors, we constructed a nomogram to determine the independent prognostic value of the cuproptosis score in HCC, and its relationship with clinical factors through uniCox and multiCox analysis ( Figure 5a ). The calibration curve demonstrated excellent agreement between the observed and predicted values of the nomogram ( Figure 5b ). To further validate the cuproptosis prognosis model, we used 259 of 517 HCC samples as a training set and 258 HCC samples as a test set in survival analysis and Cox regression. The samples were divided into low-risk and high-risk groups according to risk score. Survival analysis revealed that the high-risk group had poorer survival than the low-risk group in training and test set ( Figure 5c ). Kaplan-Meier curves consistently showed consistent prognostic effects in both datasets. The average AUC values for predicting 1-, 3- and 5-year prognosis in the training set were 0.774, 0.778 and 0.774, respectively, whereas those in the test set were 0.688, 0.658 and 0.638, respectively. ( Figure 5d ). In two different sets, the training and test set also had the big area under the ROC curve (AUC), thus demonstrating the prognostic efficiency of the risk model. The distribution of disease risk scores and the survival between groups is illustrated in Figure 5e .

Survival evaluation, on the basis of CRGs in the training and test set.
(a, b) Comprehensive nomograms and calibration maps for predicting 1-, 3- and 5-year survival of patients with HCC. (c) Kaplan-Meier survival curves showing survival rates with high or low risk scores in the training and test groups. (d, e) ROC curves, 1-, 3- and 5-year survival profiles and heat maps of prognostic molecules for the training and testing sets.
3.6 The immune landscape of cuproptosis subtypes
The R package CIBERSORT was used to analyze the proportions of tumor immune infiltrating subsets, and 21 immune cell profiles in HCC samples were constructed ( Figure 6a ). A heat map was generated to illustrate the correlations among these 21 immune cell types ( Figure 6b ). We investigated TME cell infiltration on the basis of two constructed clusters. CD4 T cells, dendritic cells, eosinophils, natural killer cells, natural killer T cells, regulatory T cells, THC, TH1, TH2 and TH17 T cells were more significantly clustered in cluster B ( Figure 6c ). In addition, a tumor-associated stromal score and an immune score representing the level of immune infiltration were obtained with the ESTIMATE algorithm. These scores were combined to generate an index called the "estimated score" to infer tumor purity. The estimated scores, stromal scores and immune scores were lower in the high-risk group than the low-risk group ( Figure 7a ). However, these scores did not demonstrate any significant correlation with patient clinical characteristics (sex, age or stage) ( Figure S2 ). We hypothesized that CRGs might influence tumorigenesis in some other way; therefore, we investigated the correlation between CRGs and inflammatory infiltration. Neutrophils, and M0 and M2 macrophages, were significantly positively correlated with the risk score, whereas B cells, CD4 T cells, CD8 T cells and regulatory T cells displayed a negative correlation ( Figure 7c ). We subsequently explored the correlations of immune cells and CRGs with favorable prognosis, and generated a heat map ( Figure 7d ). The findings unveiled the involvement of CRGs in the regulation of immune infiltration.
3.7 Characteristics of the cuproptosis score with respect to tumor mutations
Subsequently, we used the maftools software package to comprehensively analyze the distribution of somatic mutations between CRGs in the high-risk and low-risk groups in patients with HCC in TCGA ( Figures 8a, b ). To further explore somatic mutations among patients with HCC in the high-risk and low-risk groups, we used the R maftools package for analysis. The mutation rate of the CTNNB1 gene was higher in the high-risk group than the low-risk group (25% vs 26%, respectively). Additionally, TMB and RNA quantitative analysis confirmed that CRGs in the high-risk group were significantly positively correlated with TMB and RNA levels ( Figure 8c, d ).

Characteristics of CRG modification in tumor somatic mutation.
(a) Waterfall-diagram visualization of tumor somatic mutations in low- and high-risk groups. (b) Statistical differences in TMB between the high- and low-risk groups, according to the Wilcoxon Rank Sum test. (c) Scatter plot showing a significant positive correlation between risk score and TMB. (d) Scatter plots showing a significant positive correlation between risk score and RNAs.
4. DISCUSSION
Unrestrained proliferation is a hallmark of tumor cells, which evade growth inhibition by resisting cell death and evading immune-mediated killing. Induction of programmed cell death is an effective strategy in tumor therapy [20]. Studies have shown that programmed cell death has several modes, including apoptosis, neuroapoptosis, cell necrosis and ferroptosis. Excess copper induces cell death, similarly to iron [14]. Recently, the term cuproplasia has been proposed to describe the phenotypic manifestation of this particular tumor type, characterized by copper-dependent cellular proliferation [21]. However, the exact mechanism remains unclear [22]. Tsvetkov et al. have found that cell death is induced when copper is loaded on elesclomol (a copper ionophore) [22]. Those findings suggest a new potential therapeutic approach for treating tumors. In our study, we explored the expression profiles of 19 CRGs in HCC tissues. The reliability of clustering was demonstrated through PCA by construction of two cuproptosis clusters with significantly different immune infiltration and FRG features. Cuproptosis cluster B exhibited high immune cell infiltration but interestingly contained many innate immune cells, such as dendritic cells, eosinophils, natural killer cells, natural killer T cells and regulatory T cells. Copper is essential for the development and maintenance of immune function. Several findings have suggested that copper deficiency disrupts immune function, and an increased copper ion content inhibits the antigen presentation from dendritic cells to T cells, and the proliferation of T cells. Increasing copper concentrations have been found to promote the killing effect of macrophages through the Fenton reaction, thereby producing ROS [23]. By inhibiting the phosphorylation of STAT3 and EGFR, copper chelators facilitate the ubiquitination of PD-L1 and significantly enhance the ability of CD8 T cells and natural killer cells to suppress tumor growth [24]. In contrast, CRG-based risk models have revealed significant correlations among neutrophils, macrophages, B cells, CD4 T cells and CD8 T cells. Clinical evidence has demonstrated significantly higher serum copper levels among patients with HCC than those with chronic hepatitis, and the copper concentration is closely associated with both the incidence and progression of HCC [25]. In addition, the expression of mammalian ferredoxin 1 (FDX1) is regulated by adrenomedullin, which effectively inhibits copper-induced cell death and promotes tumor growth. Moreover, the critical role of copper-mediated cell death in tumor progression and chemotherapy resistance has been demonstrated, on the basis of the enhancement of sunitinib resistance observed in clear cell renal cell carcinoma. Therefore, cuproptosis is closely associated with tumors and affects tumor development through the tumor immune microenvironment. However, further validation is required to substantiate the role of CRGs in regulating therapeutic interventions and tumor immunity [26].
We identified three clusters of DEGs associated with copper-induced mortality, according to analysis in the R package. The prognostic analysis of these DEG clusters was consistent with the survival probabilities of the CRG cluster. We demonstrated the roles of alterations in cuproptosis in the prognostication and diagnosis of HCC. Therefore, on the basis of prognostic characteristics, we explored the prognostic and clinical value of the copper mortality score in HCC. Studies have demonstrated that CRG signatures have substantial prognostic value in assessing survival outcomes among patients with HCC. In addition, the prognostic ability of the model was evaluated with training and test datasets, and the two datasets had supported a good prognostic results. Our results also highlighted that CRGs may serve as a valuable prognostic indicator for HCC.
However, further improvements are required. The analysis of the cuproptosis prognostic model in this study was based on public databases. Because of the limited availability of clinical samples, some deviations might exist in the clinical data. In addition, although we observed interactions between CRGs and immune cells, the mechanism underlying these phenomena remains unclear and must be further verified through in vitro and in vivo experiments.
Nevertheless, our study demonstrated that the CRG model exhibited robust prognostic features for HCC and was associated with immune infiltration. Therefore, this model has potential as an indicator for predicting HCC survival and prognosis.